

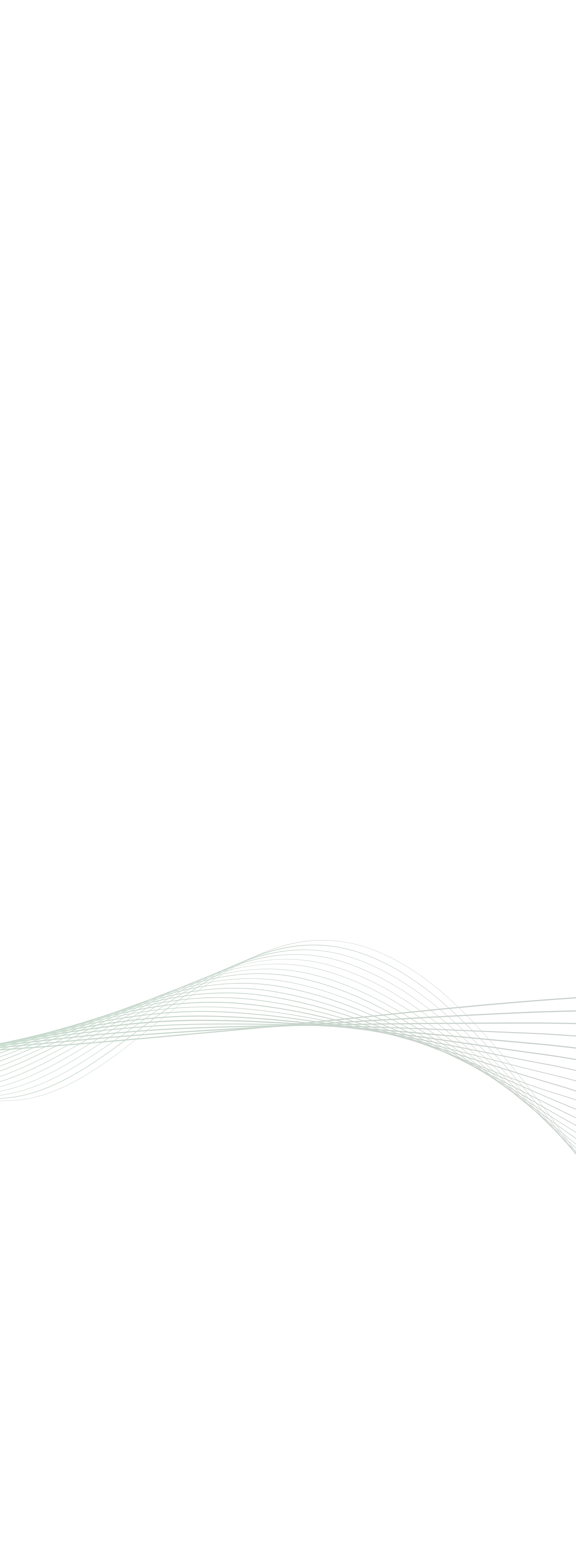
For long, everything and anything about AI has been cocooned inside deep technological workflows and engineering frameworks. It is hard for a typical business user to deduce the rationale behind how different AI models built by different organizations are driving decision-making and delivering outcomes. In most cases, users were left unaware of the specific reasoning that AI models use to interpret and generate outcomes. But it is slowly changing.
The leap towards AI transparency
It is hard to miss the growing trend amongst tech companies to focus on assuring transparency in their AI initiatives. The examples are aplenty. For one, we saw Adobe assuring openness and transparency for the data used to train AI models for its Firefly generative AI service. Another major example is Salesforce mandating notifications to users when answers by its AI to queries made by users are provided without 100% surety.
It is not just the big names in the tech industry that are actively pursuing goals of transparency in what they use to train and build their AI, but also the wider business community globally.
Why is there an urgent need for AI transparency
A recent study by McKinsey showed that nearly 92 % of businesses plan to increase their AI spending in the next 3 years and that AI can unlock long-term corporate opportunities worth over USD 4.4 trillion. AI is a mainstream technology that every organization has already embraced or will embrace soon into its core operational fabric. From healthcare, to finance and even power & utility sectors, AI is used to bring operational efficiency, drive new growth opportunities and revenue streams, and above all accelerate decision-making in critical scenarios.
With so many critical use cases coming into the big-picture embrace of AI, it is important to ensure that there are no biases or inaccuracies in the AI models being used. Fairness and accountability must be guaranteed for every possible transaction carried out by or for AI initiatives. In other words, the AI models used, and outcomes generated must be explainable and interpretable.
On a broader perspective, 3 key factors make AI explainability important:
Building trust in AI-driven decisions
In high-risk sectors like finance, AI-driven decisions can have long-term implications if outcomes are not trustful. For example, a potential loan applicant whose application is rejected by an AI-based credit scoring system would require answers as to why his or her application was rejected. The underlying AI scoring model should provide a fair understanding of how scores were calculated and what factors worked against the candidate. In the absence of clarity, the decision may lead to lawsuits and loss of reputation for the bank for the mishap.
AI security & regulatory compliance
AI models work by consuming a tremendous amount of data insights both during the training phase as well as in their operational phase. When pushed into operations across civilian use cases, enterprises must ensure that their data workflows powering AI models work in compliance with regulatory frameworks and mandates like GDPR, AI Act, and NIST AI RMF. These compliance frameworks require the AI models used in digital services to have a high degree of explainability and can potentially fine companies heavily if they fail to justify AI-powered decisions with solid rational context.
Bias & ethical considerations
In alignment with principles of diversity, inclusion, and fairness outlined by government bodies in countries across the world, digital services that serve the critical needs of the masses must ensure that every autonomous decision they make is free from bias and conform to standards of ethical conduct recommended for similar scenarios. Explainability of AI helps to ensure that such bias is eliminated at all times of making critical decisions.
How can AI models be made more explainable?
How can one make AI models and their generated outputs more explainable? Considering that AI models account for numerous data patterns and behaviors while making decisions, there are quite a few ways to make it more explainable.
To start, the model used can be inherently explainable like decision trees, or rule-based systems which have sufficient explainability on their own when it comes to decision-making. In scenarios where complex models need to be leveraged, enterprises can go in for Post-Hoc explainability methods like SHAP or LIME or Feature Importance Analysis. Additionally, they can leverage visual and interactive AI explainability methods for highly complex use cases. In such scenarios, heat and saliency maps, or attention mechanisms can provide better model transparency.
AI models can improve explainability by providing citations for the generated content. The citations are also validated by AI models before being shared with the users.
A major step towards improving AI transparency will be the setting up of an AI governance ecosystem that vets and validates AI outputs frequently. The governance committee formed must have human resources that randomly review the model outcomes and ensure that the learning curve used for training the model supports explainability of the outcomes as well.
The future of AI transparency
Embracing techniques to improve AI transparency is only the beginning of a race for businesses to bring higher levels of trust and security to their AI models. With rapid AI adoption happening across critical sectors like healthcare, finance, and even the military, the need of the hour will shift from transparency to accountability. AI models will have to predict outcomes or confidently make decisions since they will eventually be held accountable for further actions stemming from the output.
Weaving the essence of transparency into every operational element of AI initiatives will be key in preparing a business to transition into one that provides superior AI-powered digital experiences without bias. However, such a journey requires strategic oversight and a deep understanding of how transparency mandates impact AI decision frameworks. This is where having a technology partner like Xoriant can help enterprises win their AI transparency race with confidence. Get in touch with us to know more.